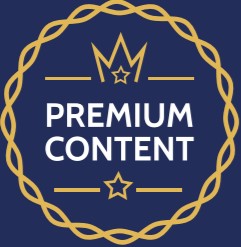
Digital Content
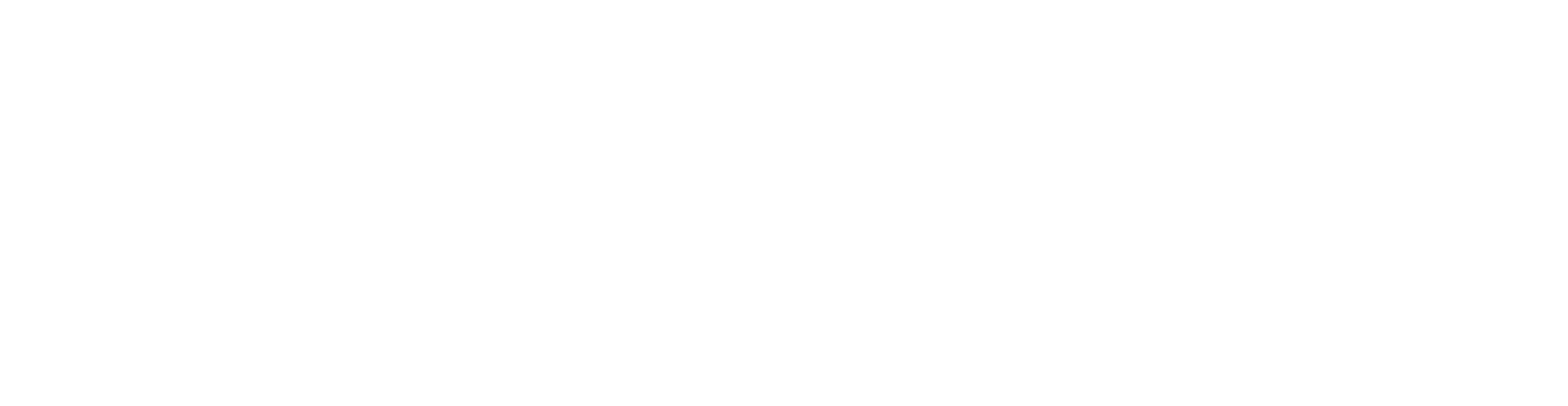
- Unlimited access to peer-contribution articles and insights
- Global research and market intelligence reports
- Discover iNFRont Magazine, an NFR publication
- Panel discussion and presentation recordings
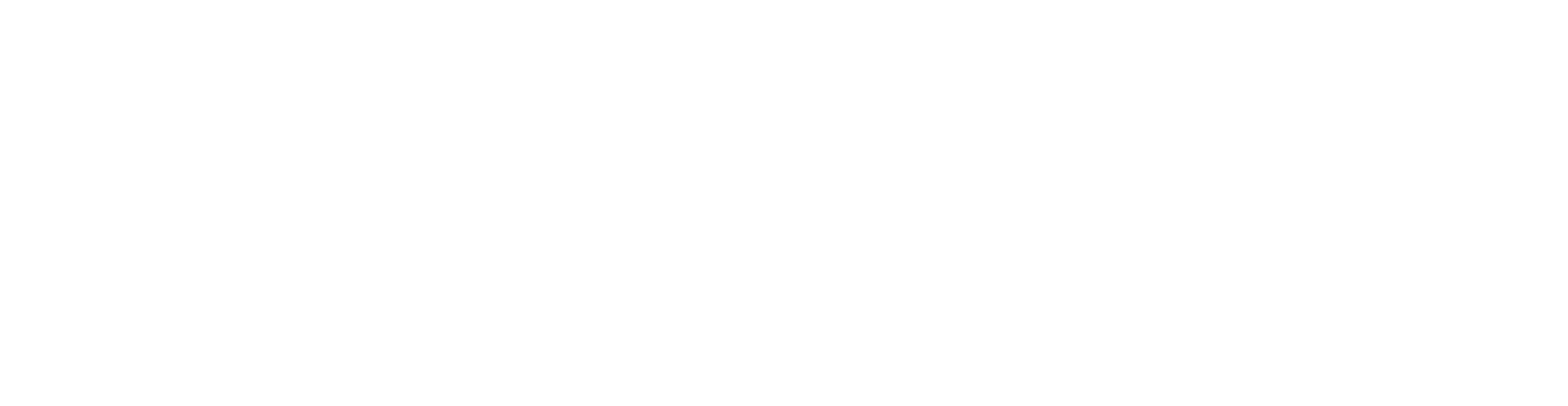
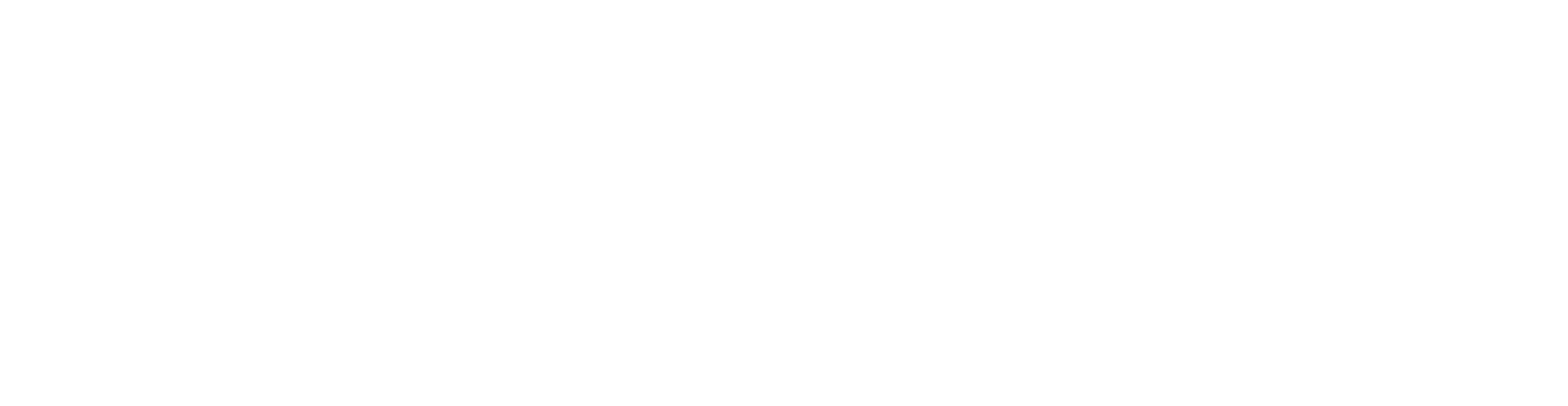
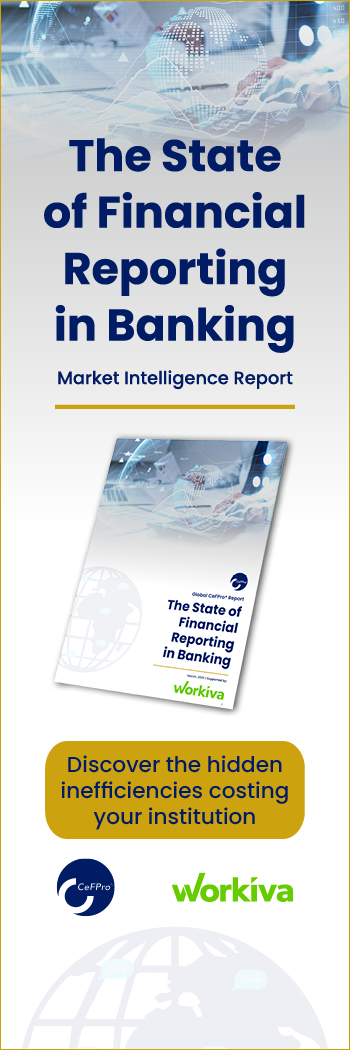
Presentation
Chandrakant Maheshwari delivers a compelling case study on how transformers, a powerful AI model architecture, can be leveraged for financial crime detection. He begins by demystifying the concept of self-attention with a classroom analogy: four students, each with different domain expertise, answer a biology question. The teacher assigns weight to each response based on relevance and forms a final, weighted answer. This mirrors how transformers evaluate input—through query, key, and value matrices—to form intelligent outputs.
Moving from theory to application, Chandrakant maps this metaphor directly to the self-attention mechanism, showing how it dynamically identifies and weighs information based on contextual relevance. From this foundation, he explains how stacking self-attention layers gives rise to transformers, which excel at identifying nuanced, contextual patterns.
He emphasizes that fraud detection in financial services requires more than static rules—it needs a model that understands behavior over time. Unlike traditional systems that evaluate isolated transactions, transformers ingest entire sequences, identifying anomalies based on evolving relationships between transactions.
Finally, a case study brings the theory to life. Chandrakant walks through an example with six transactions, transforming raw features into embedded representations. He outlines how transformers use query (the transaction’s intent), key (its characteristics), and value (its contribution to fraud detection) to flag suspicious behavior. This approach allows for real-time, high-accuracy fraud detection by treating transactions like interconnected language—where meaning emerges from relationships.
Chandrakant Maheshwari is a seasoned expert in Financial Risk Management with over 20 years of experience specializing in Model Risk Management Financial Crimes and AML Compliance. He is a thought leader in integrating AI technologies into risk management frameworks. Chandrakant is an advocate for using Generative AI to enhance model validation and risk assessment processes driving innovation while maintaining robust regulatory compliance. A published author and frequent speaker at industry conferences he is passionate about mentoring the next generation of risk professionals and advancing the practical applications of AI in finance.