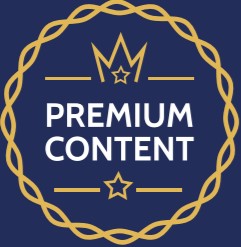
Digital Content
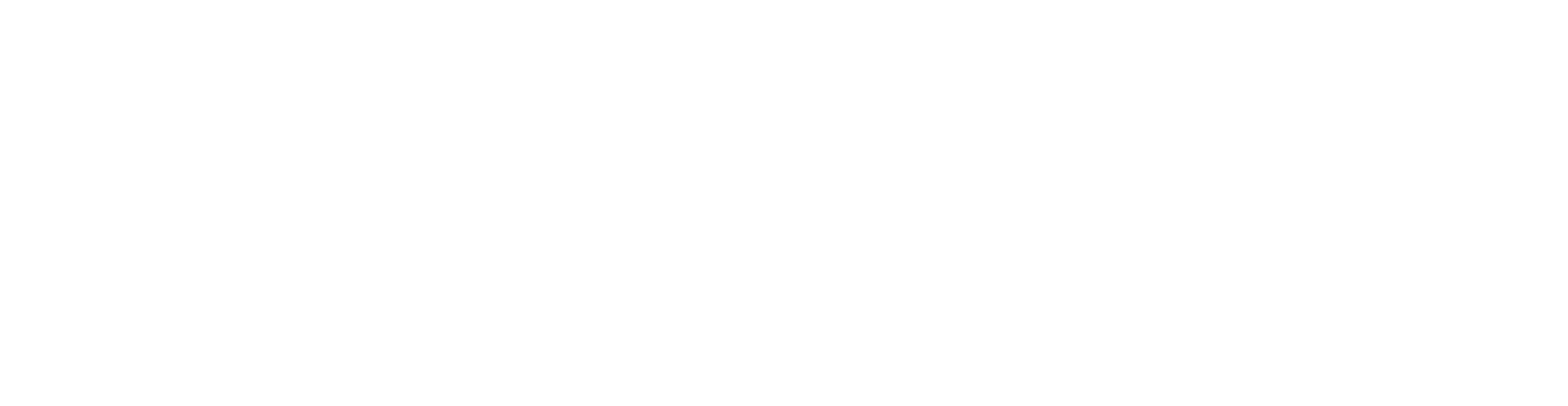
- Unlimited access to peer-contribution articles and insights
- Global research and market intelligence reports
- Discover iNFRont Magazine, an NFR publication
- Panel discussion and presentation recordings
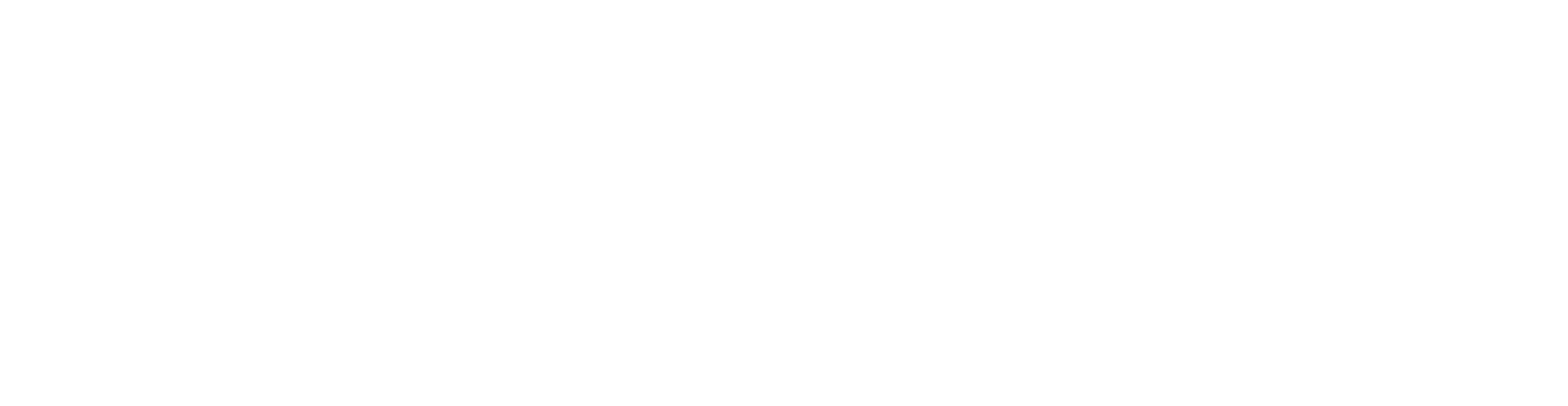
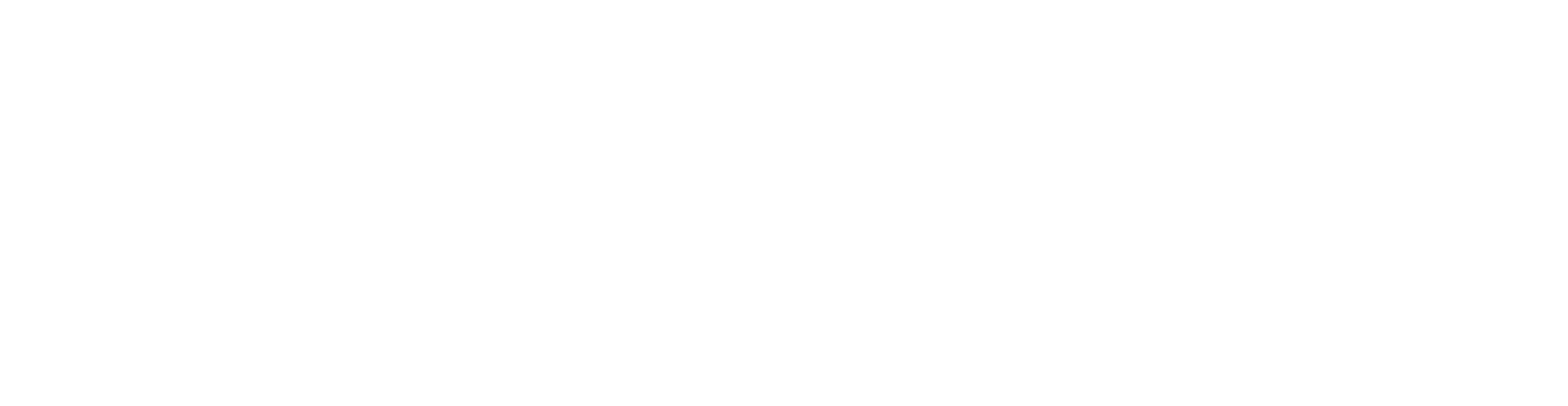
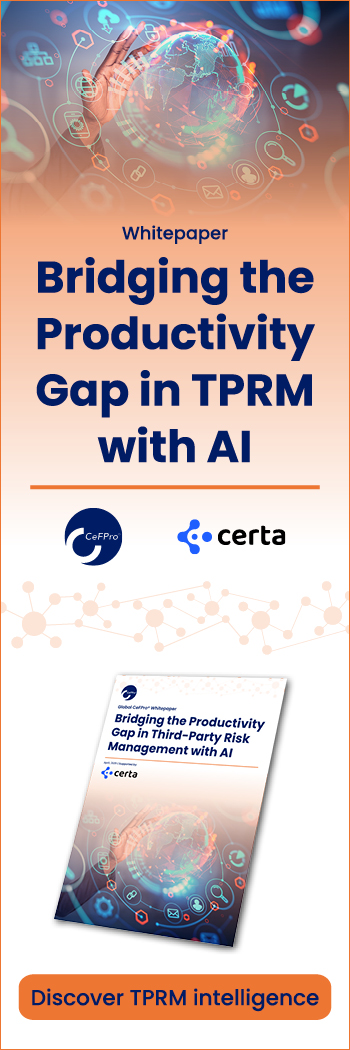
Video
In this insightful conversation, Chris Smigielski, Director of Model Risk Management at RFS Bank, shares how AI is reshaping risk functions in the banking sector. The most impactful uses of AI today are in fraud monitoring, information security, and credit scoring. AI models outperform traditional methods by rapidly identifying patterns that are nearly invisible to human analysts, offering a level of efficiency and precision that is transforming these critical areas.
However, Smigielski stresses that many organizations struggle not with the idea of AI, but with its implementation. AI is not just another tech tool—it introduces entirely new risks, requiring a collaborative approach that spans IT, compliance, model risk management, and business lines. A successful AI strategy demands a pre-built control framework and a cross-functional team, rather than ad-hoc responses to challenges that arise during deployment.
One of the most debated issues in AI governance is how to classify AI systems—are they models or tools? Smigielski argues it depends on their impact on decision-making and financials. His institution uses a dual-inventory system, categorizing AI-based models separately from AI tools, each governed by its own set of policies and procedures within a broader AI center of excellence.
Smigielski also warns of risks like model drift, using an example of a machine learning model that degraded over time due to a lack of new training data. Annual reviews uncovered the issue, reinforcing the need for continuous monitoring and robust model lifecycle management.
Looking ahead, Smigielski predicts a future where nearly every application will include AI components. As agentic AI becomes more common, he envisions a standardized framework that manages both models and tools, along with AI systems designed to monitor other AI. It’s a shift toward a more sophisticated, interconnected ecosystem—one where risk management must evolve in tandem with technological advancement.
With over 30 years of financial services industry experience, Chris has an in-depth knowledge of model risk management, model governance, model validation, financial model development, Asset Liability Management, and team development. Chris is currently the Director of Model Risk Management and a member of the AI Center of Excellence governing the use of AI at Arvest Bank. Chris was previously Vice President, Director of Model Risk Management at TIAA Bank for five years. His experience includes leadership roles at Diebold and Fiserv, where he consulted with financial institutions nationally and internationally to design and implement financial strategies to maximize productivity and growth, as well as Asset/Liability Management and quantitative analysis at HSBC and First Niagara Banks.
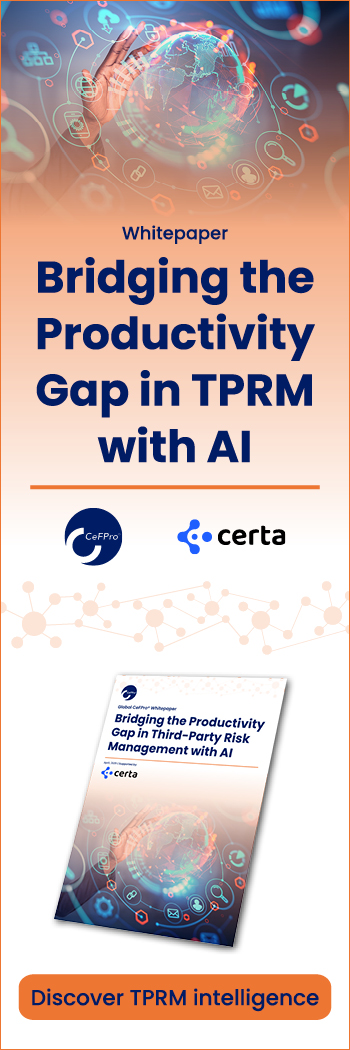