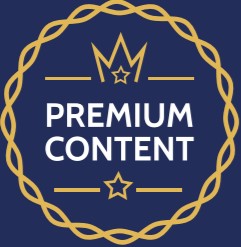
Digital Content
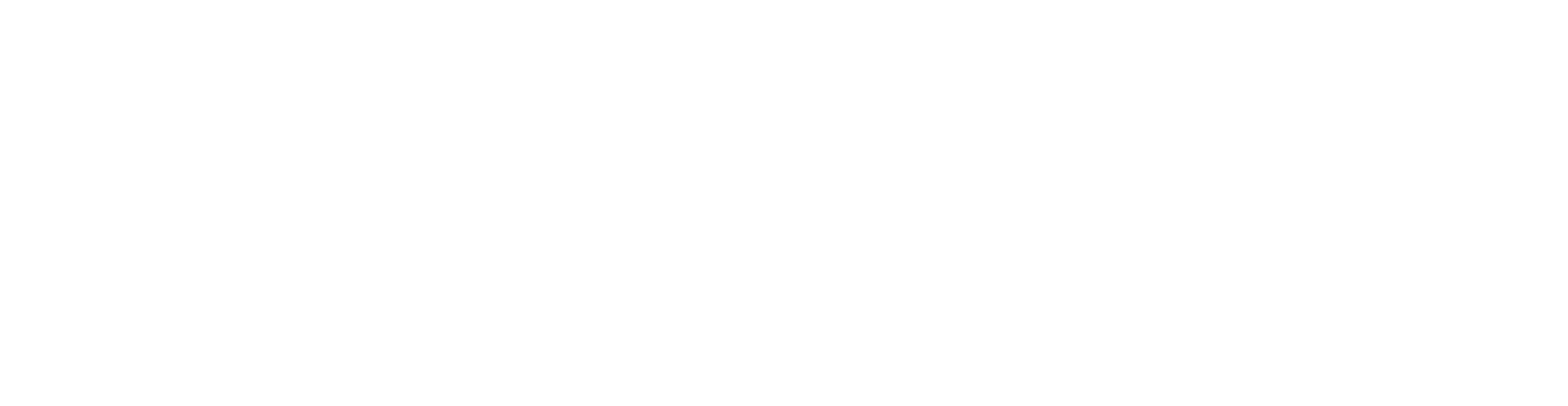
- Unlimited access to peer-contribution articles and insights
- Global research and market intelligence reports
- Discover Connect Magazine, a monthly publication
- Panel discussion and presentation recordings
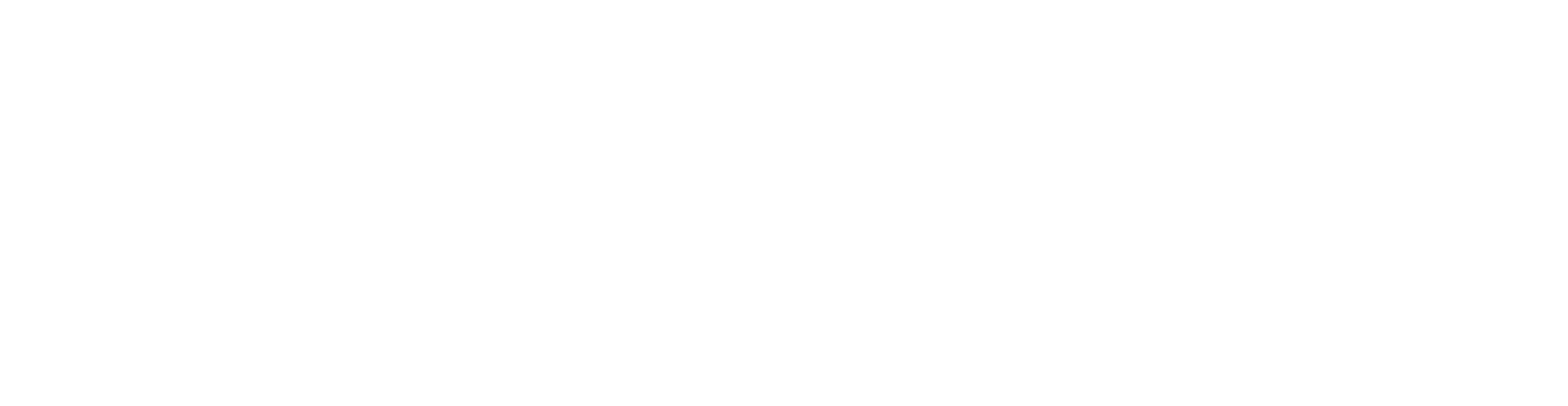
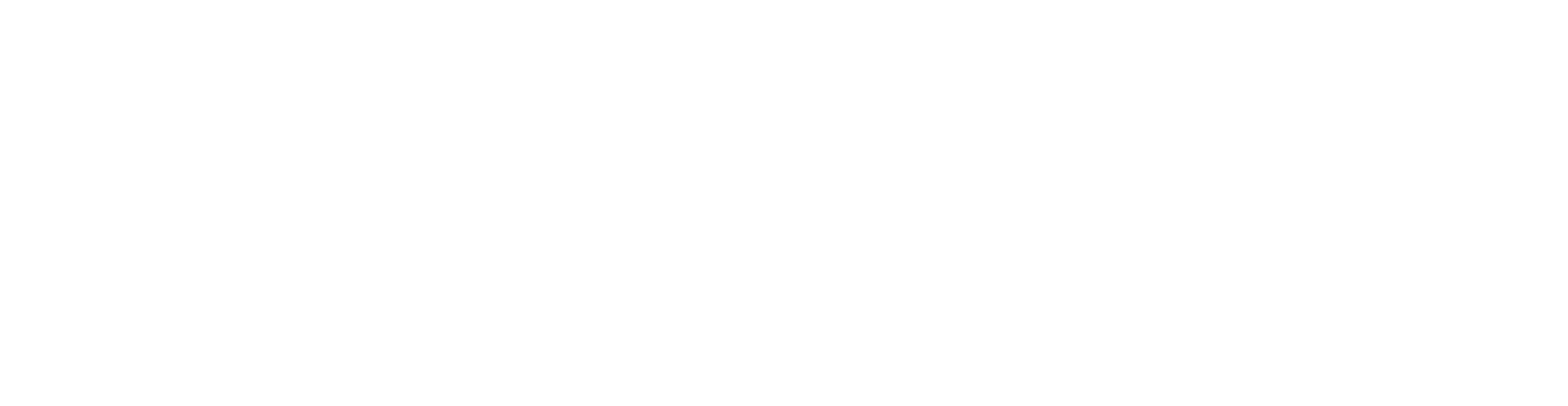
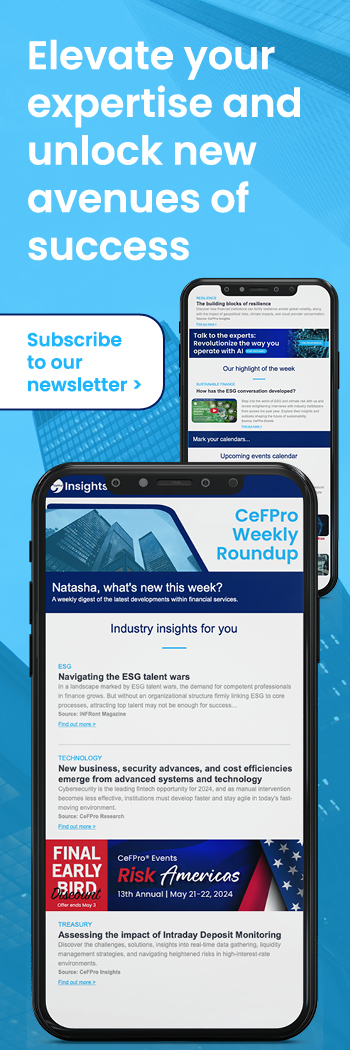
Video
Banks are increasingly integrating AI and machine learning into their risk management frameworks, but this shift brings both opportunities and challenges. To enhance model risk management, financial institutions are developing specialized assessment frameworks that account for the complexities of AI-driven models.
However, the adoption of these techniques requires significant upskilling of internal teams, ensuring that expertise remains within the organization rather than relying solely on external support. Mitigating risks associated with AI is a priority, with banks implementing safeguards such as closed knowledge bases and mandatory human oversight to maintain control over model outputs.
As AI-driven models are used for both managerial and regulatory purposes, institutions must navigate strict validation processes, balancing innovation with compliance to gain approval from regulators and top management.
Rita is “Head of Internal Validation and Controls” in Group CRO Area of Intesa Sanpaolo with responsibility on Internal Validation, Model Risk Management and II level credit controls and data governance controls at group level; she has been 15 years in Financial Risk Management as Head of Market and Counterparty Risk Internal Models. Main achievements in current role have been: Creation of a Group Model Risk Management Unit, Definition of MRM Group Policy, Model Risk Assessment Methodology, Model Tiering Methodology, Model Risk Appetite, Top Management Model Risk Reporting, Independent Validation for regulatory and managerial models, Evolution of Internal Validation on regular assessment of Data Governance Framework according to ECB guide on RDARR.
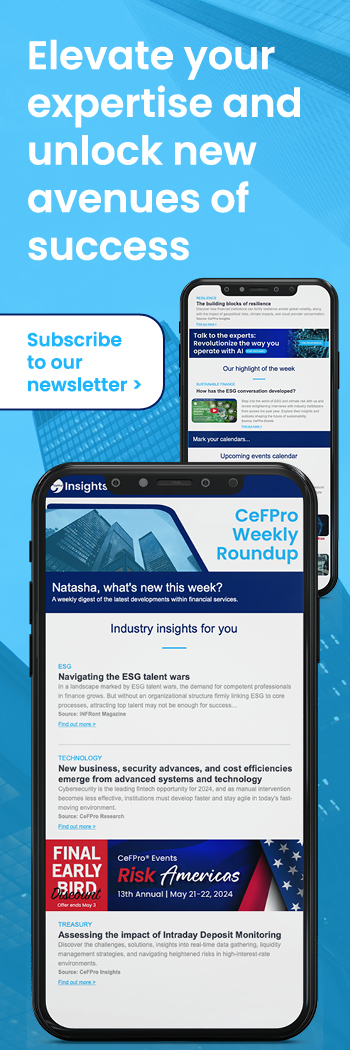